Climate by design
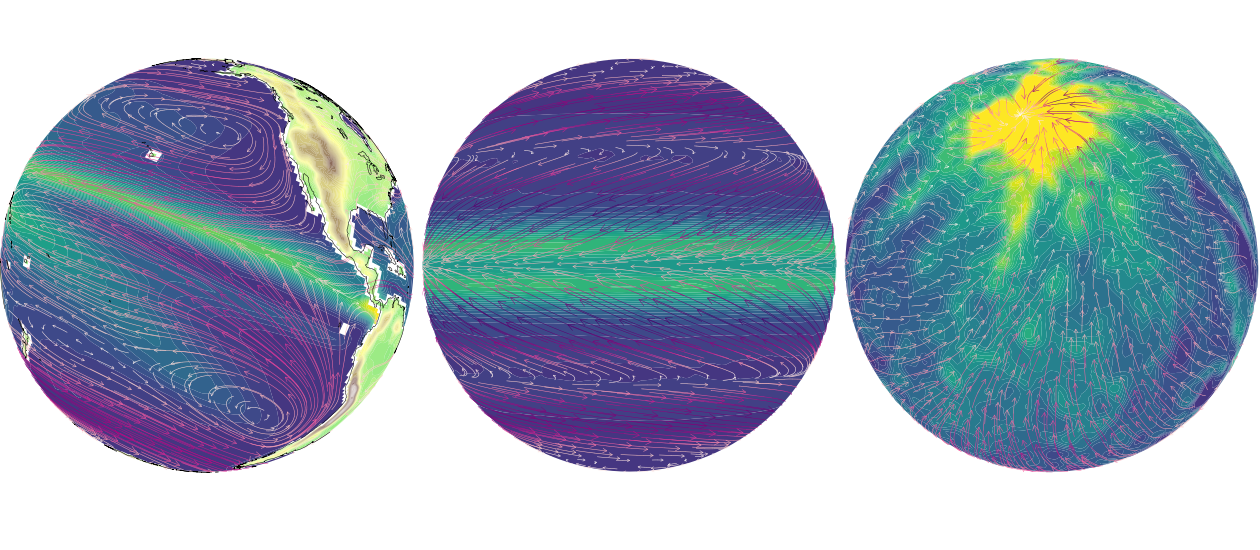
I am interested in the role that clouds play in the climate system. Clouds embody complicated, delicate interactions of atmospheric dynamics and thermodynamics, which come together to substantially alter the state of the atmosphere. Because so many scales are involved, from sub-millimeter (e.g. nucleation) to thousands of kilometers (e.g. planetary-scale waves), from seconds to years, the representation of clouds in climate models is challenging, and has remained a source of uncertainty in projections of future climate. My work uses these models to try to (1) better understand how clouds interact with the climate system and (2) to improve the representation of clouds in climate models.
Climate models provide our most comprehensive tools for understanding the climate system. In my work, I try to contrive numerical experiments with climate models that help understand both how the real world works and how the model works; occasionally those are the same thing. Wherever possible, I use satellite observations and reanalyses as measures of the real world.
Since 2009 I have been a Project Scientist in the Climate and Global Dynamics Laboratory at that National Center for Atmospheric Research in Boulder, Colorado. NCAR is largely responsible for the development of the Community Earth System Model (CESM), and its atmospheric component, CAM. This is the modeling system I use most, though I also analyze multi-model archives to compare different variations of climate models. When I started use CAM, it was in Version 3. When I joined NCAR, Version 4 was current, but Version 5 was released shortly after I started. From about 2010 to 2017, most of my work has been with CAM5. I used developmental versions of CAM5 extensively from about 2014 to 2017, partly to contribute to the development of CAM6 (released in 2017).
One approach I use is to employ methods of weather prediction to understand sources of error in climate models. Running in forecast mode allows direct comparison with observations and evaluation of process-level diagnostics. This approach also provides opportunities to examine sources of predictability in the climate system, including examination of interesting topics such as the role of coupling between the atmosphere and other climate system components in making skillful predictions. Our version of "forecast mode" was jointly developed by LLNL and NCAR in the early 2000s and became known as CAPT (now meaning Cloud Associated Parameterization Testbed). For the past several years, I am the PI of the CAPT project. I work with colleagues at NCAR and externally to maintain the CAPT framework, expand its capabilities, and apply it to test model behaviors.
The climate system is full of feedback loops, which are ultimately responsible for the chaotic nature of the system and the difficulty in predicting both weather and climate. While the CAPT approach is great for examining the fast processes in the climate system, the system soon forgets its initial state as it settles into its own climatic state. Because of the relevance to society, it is important that the model's preferred climate is as close to reality as possible, including its response to changes in the frorcing of the system. I am interested especially in how clouds and their associated phenomena respond to changes in forcing, which is at the heart of the cloud feedback problem. This includes feedbacks between clouds and their environment on short timescales and also the classic cloud-climate feedback which has plagued climate sensitivity estimates for decades.
Clouds are parameterized in conventional climate models. This means that the cloud cover and other cloud properties are calculated using inferred relationships between the clouds and their environment. Over the years, these schemes have become more elaborate, and quantitatively better at representing clouds. As the models have become more sophisticated, understanding their behavior has become more complicated. In an attempt to distill the model behavior that emerges from the chaotic system, I often run the model in exotic configurations. My favorite is the aquaplanet, which is a water-covered, Earth-like planet that (typically) has no seasons and usually lacks some important physics like interactive oceans and sea-ice. In these idealized configurations, I ask the same questions that are important in the realistic simulations, like, "how do clouds change when the planet warms?" I compare the idealized responses to the realistic configurations to see what aspects of the idealized configurations are present, and whether these can help to understand basic physics of the climate system (or errors in the climate model).